Decarbonizing electricity systems will require high levels of flexibility from loads that can be leveraged as flexible solutions at both the bulk power system and distribution system levels.
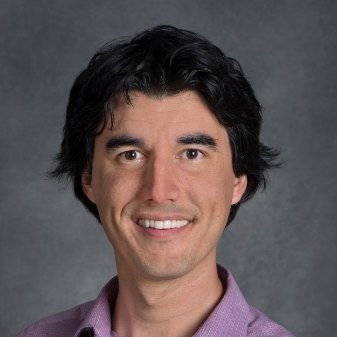
Juan Pablo “JP” Carvallo
Traditional demand response (DR) programs such as direct load control will continue to play an important role, but price-based DR programs, implemented through time-based rates, are a relatively untapped source of flexibility in the United States.
What Is Price-Based Demand Response?
Price-based DR is simply a retail rate designed to induce a short-term change in customer energy use by varying the price of electricity for a given period of time or event. Typically, the price is higher during some blocks of time and lower in other blocks, inducing a shift in the timing of consumption. The traditional intent of this strategy is to discourage consumption during specific times of the day or year in which the system is stressed and running out of resources. But with increasing growth in variable renewable resources, some utilities are adopting rates that encourage consumption during times of high wind and solar production.
One of the most common price-based DR strategies for residential customers is time-of-use (TOU) rates. On-peak prices are higher and off-peak prices are lower than the standard basic service rate. The price schedule is fixed and predefined, based on season, day of the week, and time of day. On the other end of the spectrum is real-time pricing (RTP) in which prices vary hourly, tracking the wholesale market or locational marginal price. Other rates used to nudge customers to reduce consumption during specific events through higher prices or incentives are critical peak pricing (CPP), variable peak pricing (VPP), and critical peak rebate (CPR).
How Do You Estimate the Impacts of Price-Based DR in Integrated Resource Planning?
There are two parts to this question: (1) estimating the impacts of price-based DR—or any type of DR—typically involves modeling using the framework in Figure 1, and (2) realizing the impacts means implementing price-based DR in a way that actually works for customers and the power system. The benefits of price-based DR can be realized in wholesale markets or in integrated resource planning (IRP), which is where the framework below is typically applied and where we will focus the rest of this blog.

Figure 1: Framework to Model the Impacts of Demand Response Source: Lawrence Berkeley National Laboratory, used with permission.
In recent work by Berkeley Lab, Lisa Schwartz and I critically analyzed how a dozen utilities modeled and integrated price-based DR as a demand-side resource in their latest IRPs. (We also reviewed nascent efforts to consider price-based DR in distribution system planning.) We used this framework to understand how utilities modeled various components, what values they used, and why.
What Did We Find?
We found lots of things! Seriously, perhaps the most interesting finding is the wide variation in quantitative assumptions in different parts of the framework, such as the cost of program set-up, achievable potential reduction in peak load, and assumed load reductions achieved. We also found that most assumptions seem to lack rigorous support, with significant opportunities for data-driven approaches to refine values and evolve them over time. You will have to read the technical brief to learn about all the details, because in this post I focus on one example—enrollment or participation rates.
Participation rates describe the utility’s estimate of what share of their customers would switch to a given price-based DR option. Table 1 provides data for the eight utilities in our sample that reported participation rates for three residential programs and three commercial/industrial (C&I) customer programs. The first thing that pops out is the utility that reports opt-in and opt-out values. Opt-in assumes the customer has to intentionally switch to the new rate; opt-out assumes the customer will be defaulted into the new rate and has to take intentional action to return to their original rate or select an alternative rate (if the utility provides these options).
The variation in expected uptake for DR options—especially TOU rates—across the utilities in our sample is staggering. For comparison, in 2019 less than 2% of U.S. residential customers were enrolled in a TOU program (although half of the customers did not have the chance to enroll in such a rate), but this number grew to about 10% in 2024. The potential of opt-out approaches to achieve massive uptake levels is formidable, considering 85% to 92% opt-out adoption rates reported in TOU pilot programs.
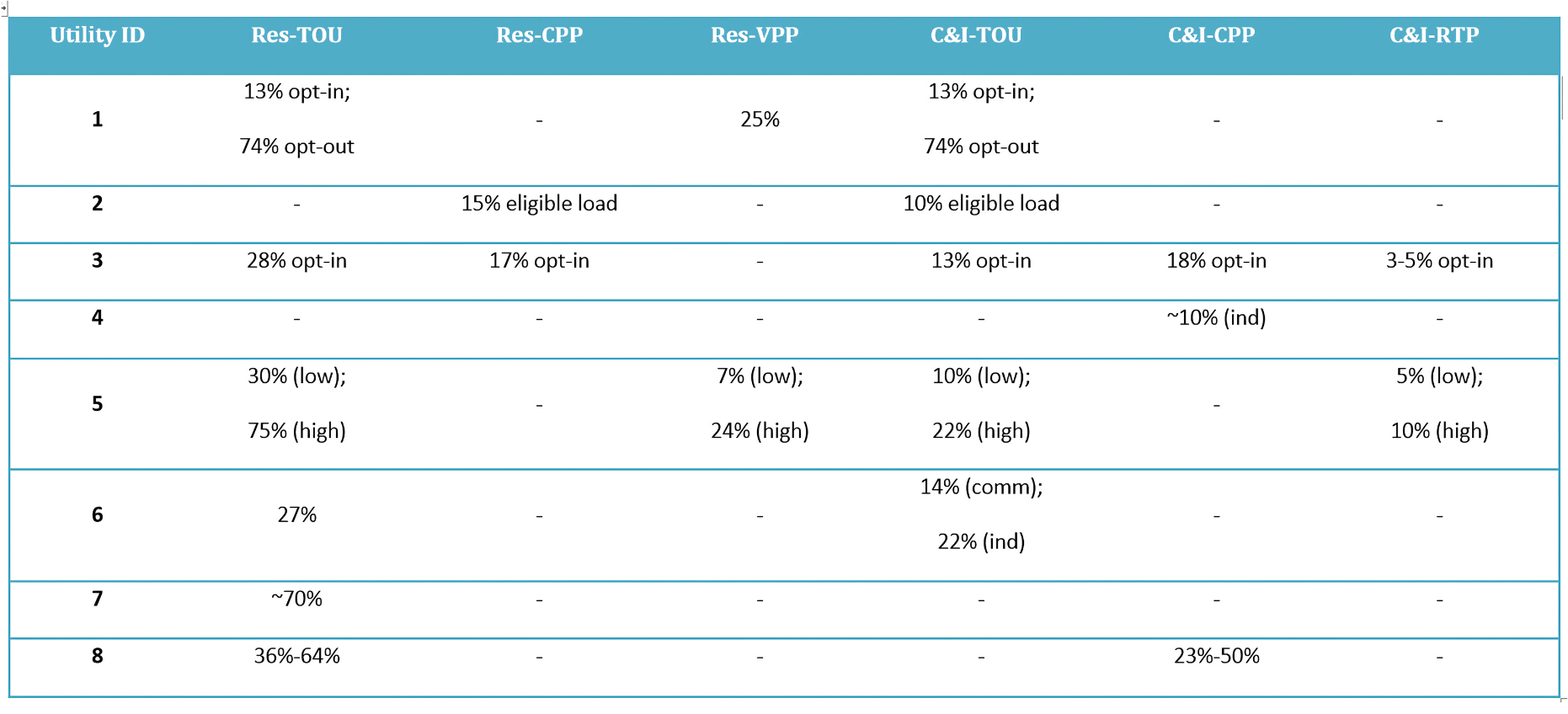
Table 1: Expected Participation Rate During the IRP Study Period, by Customer Class and Rate Type, for All Reporting Utilities in our Sample Source: Lawrence Berkeley National Laboratory, used with permission.
The median low value of assumed participation rates during the IRP study period (typically 10 to 20 years) across the eight utilities is closer to 30%, which is still three times as high as customers enrolled in TOU rates today. It is possible that utilities that file IRPs are more active in incentivizing customers to switch to TOU rates. However, it is more likely that these values suggest that the utilities expect growth in customers enrolled in TOU rates will continue. Unfortunately, the IRPs reviewed for our study say little about the assumed rate design, rationale for assumed adoption rates for time-varying pricing, how fast assumed adoption rates will be achieved, and assumptions for opt-in vs. opt-out approaches. Interestingly, the uptake of any time-varying rate for C&I customers is expected to remain quite low over the IRP study period. It is possible that these values reflect incremental rather than aggregate penetrations. In addition, C&I customers have historically been much more active users of time-varying rates, so the utility’s pool of customers in this market segment not already participating in such a rate is typically smaller compared to residential customers.
The utilities’ assumed adoption levels suggest that TOU is likely the next big step in time-varying rates, but that rates that respond dynamically to price signals are still far from being implemented at scale. A combination of granular data, automatic controls, customer dashboards, awareness, and regulatory requirements may nudge customers to accept and adopt these sophisticated rates.
To learn more, see JP Carvallo and Lisa Schwartz (2023), Berkeley Lab, The use of price-based demand response as a resource in electricity system planning
Juan Pablo “JP” Carvallo
Research Scientist
Lawrence Berkeley National Laboratory
Leave a Reply